In a previous post, I tried to resolve how to find if an exercise is executed in a correct form, using OpenPose to gather exercise data and Dynamic Time Warping to compare the exercise execution with a correct one as a baseline.
This time I added the following other components to greatly enhance the overall experience:
- The awesome Face Recognition library (https://github.com/ageitgey/face_recognition), used to perform face recognition and load an appropriate workout
- The awesome Speech Recognition (https://github.com/Uberi/speech_recognition), used to process user’s answer
- The awesome Google Google Text-to-Speech (https://github.com/pndurette/gTTS), used to talk and guide the user
This is a graphical representation of the workflow
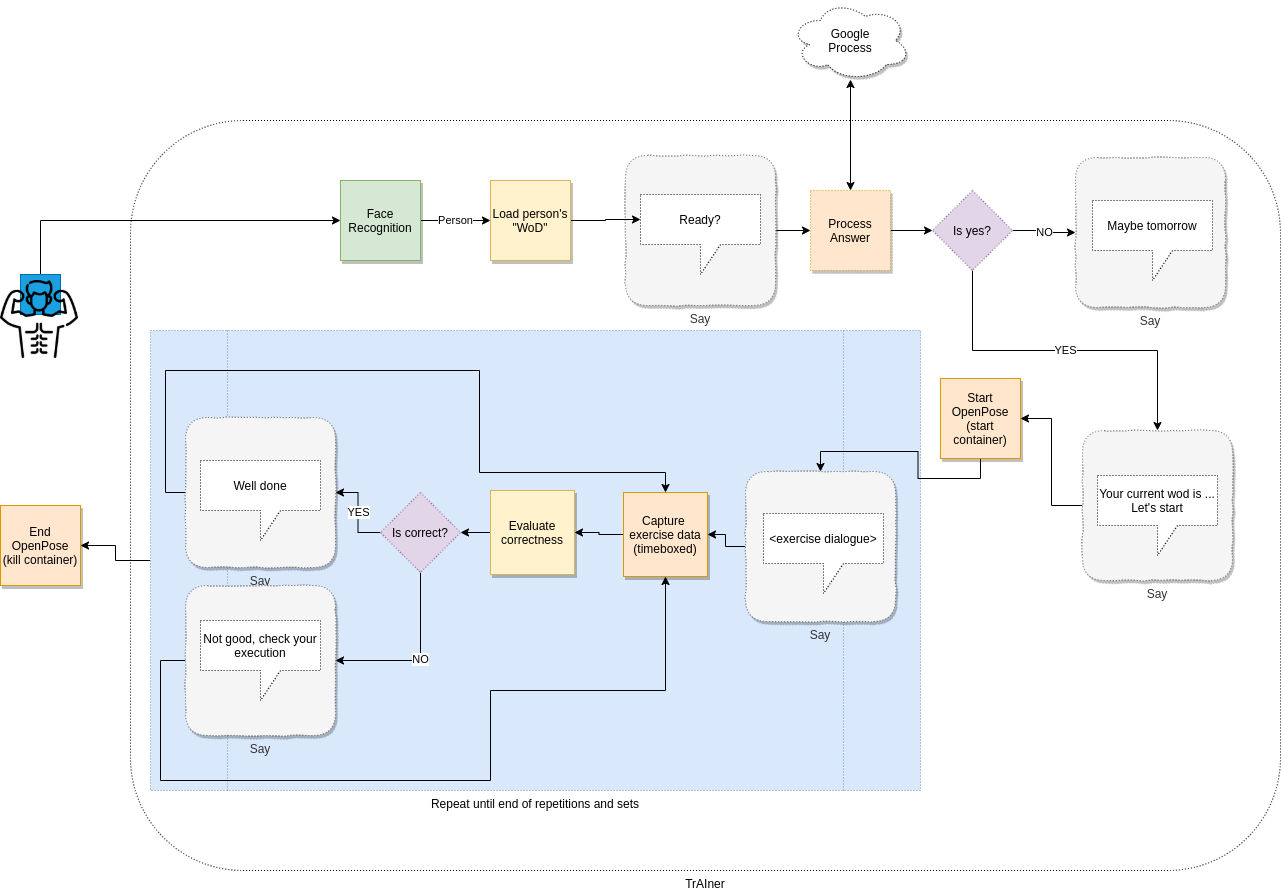
I’m still using the “dockerized” version of OpenPose (https://github.com/garyfeng/docker-openpose) — installing locally is quite long — and tweaking some parameters is possible to achieve good fps too.
The experience is quite straightforward:
- You enter in the exercise area and the Face Recognition component match your face, extracting a profile (just a name for now)
- The trainer asks if you’re ready to start and waits for an answer (a “yes” at least — this part is scripted but, of course, can be extended with more complex solutions)
- The trainer loads your associated “wod” — workout of the day — with the exercises to perform (just one for now)
- You perform exercise repetitions and sets while the OpenPose component records the body data
- The trainer compares the gathered data with the correct baseline, evaluating the dynamic time warping value for every part of the body and finally giving a median value.
- If the value is below a certain threshold, the exercise is considered correct and the trainer gives this feedback otherwise it warns (a generic warning for now, but should be possible to have specific hints based on which body parts were incorrect)
- Repeat until all the repetitions are done
But let’s see in action — sorry for the video editing, definitely I’m not good doing it :)
I worked hard to have a decent experience but, of course, there is a lot more to do.
For example, now the exercise estimation is timeboxed but it should be possible to train the system to understand when an exercise is started and finished, discarding other frames for the evaluation.
Moreover, this is just a unique exercise type (pullup) and with a baseline correct exercise specifically done with my body measurements, but it should be possible to perform different exercises with a generic baseline.
A more precise feedback on what was specifically wrong during execution is another interesting point to investigate.
One achieved this, it could be used for any kind of exercise (fitness, yoga, etc) and with objects too (using custom tracking object algorithms), in a standalone mode, or as an assistant to a real trainer.
This is another example showing what is possible to achieve in hours, combining different tools and libraries, something that a few years ago would have been unthinkable and that never ceases to amaze me!
I hope you enjoyed reading this (but take a look at the above video too) and thank you!
Appendix
Let’s see some code to see how it’s simple to perform face recognition and text to speech.
For the OpenPose part and exercise evaluation, just refer to my previous story appendix
Face Recognition
Just used the code available in the demo part
All the magic is in the encoding. Once a known face is encoded, then you can compare it with the frames captured by the camera
antonello_image = face_recognition.load_image_file("faces/anto.jpg")
antonello_image_encoding = face_recognition.face_encodings(antonello_image)[0]
Talk and process answer
Just saving the text to an mp3 file and then play it to say something
def say(text, language = 'en'):
speech = gTTS(text = text, lang = language, slow = False)
mp3_file = 'text.mp3'
speech.save(mp3_file)
p = vlc.MediaPlayer(mp3_file)
p.play()
time.sleep(2)
To process answers, we’re using the Google engine.
The code is super easy
def process_answer():
recognizer = sr.Recognizer()
microphone = sr.Microphone()
with microphone as source:
recognizer.adjust_for_ambient_noise(source)
audio = recognizer.listen(source)
try:
response = recognizer.recognize_google(audio)
print('Received response {}'.format(response))
except sr.RequestError:
print('Error')
except sr.UnknownValueError:
response = 'Sorry I cannot understand'
return response
Putting all together you can perform a basic dialogue
person = recognize() # using the face recognition
say('Hello ' + person + ', are you ready?')
response = process_answer()
if ('yes' in response):
say('Ok, let\'s start')
else:
say('No problem, maybe tomorrow')
---------
Sono un Coach specializzato e IT Mentor, con 25 anni di esperienza nel settore IT. Se vuoi migliorare la parte Tech della tua Azienda o migliorare te stesso/a, sono qui per supportarti. Scopriamo insieme come
Sono un Coach specializzato e IT Mentor, con 25 anni di esperienza nel settore IT. Se vuoi migliorare la parte Tech della tua Azienda o migliorare te stesso/a, sono qui per supportarti. Scopriamo insieme come